When We Forecast AGI, Do We Mean In The Lab Or In The Field?
The Onset of AGI Will Play Out Over Decades
The nuclear era began at 5:29 AM on July 16, 1945, when the Trinity device was detonated at the test site in New Mexico. Prior to that moment, nuclear weapons were just a theoretical possibility; afterwards, their practicality had been unequivocally demonstrated, with immediate military and geopolitical implications.
AGI won’t have any equivalent demarcation line. There’s not going to be a fateful moment where someone flips a switch and the AI opens its eyes, blinks, looks around, says “thank you for creating me, I’ll take things from here”, and then manifests the Singularity. The advent of AGI, like the rise of e-commerce, will be hard to pinpoint.
AGI is now near enough that when we speculate as to when it will arrive, we need to specify which point in the gradual process of “arrival” we are referring to. This is illustrated by a recent paper, Transformative AGI by 2043 is <1% likely1. The paper models the timeline of “transformative AGI”, which the authors define as follows:
…the systems in question must already exist and be deployed at scale, as opposed to technology which merely leads suggestively in that direction.
They are not speculating as to when we’ll manage to build a single AGI in a virtual lab. They are modeling the date by which human-level robots – including physical bodies – are available in mass quantities, at a cost below $25 / hour2.
Is that the right criterion? Do we “have AGI” when we (barely) know how to operate a single, disembodied human-level intelligence that occupies an entire data center? Or does it not count until you can call 1-800-BIZ-BOTS and have 1000 affordable android workers delivered to your factory first thing Monday morning?
Mapping The Emergence of AGI
When we talk about AGI timelines, we usually have some particular consequence in mind, such as “when will AI begin displacing large numbers of jobs”. To estimate the arrival of such a milestone, we need to think about the specific attributes that AGI will need to have achieved. Here are some important parameters:
Cost – how much does it cost to run an AGI? Most workers are paid between a few dollars per hour and a few hundred dollars per hour. A human-level AGI that costs $1000 / hour would be a laboratory curiosity3; at $1 / hour, it would be profoundly transformative.
Availability – do we have enough GPUs (and, where relevant, robot chassis) for a thousand AGIs? A million? A billion4?
Quality – does the AGI’s performance match a mediocre human? A highly talented specialist? Or world-class5?
Independence – can the system run entirely unsupervised, or does it occasionally need human assistance?
Speed – compared to a person, how long does it take the AGI to perform a given task? (This is related to quality, but only loosely. A mediocre writer is unlikely to ever produce a great novel, no matter how much time they are given. Also, to some extent, we may be able to trade off speed vs. quality vs. cost by adjusting parameters in the neural network architecture and implementation.)
Training status – is the system ready to tackle the wide array of economically important functions, or does it still need to be trained on specific tasks? Is it an infant, a fresh college graduate, or a seasoned worker?
Real-world applicability – does the system merely pass artificial benchmarks, or is it ready to provide utility across the range of real-world deployment scenarios? Does it incorporate the tacit knowledge, interface modalities, and other messy details needed to operate outside of a lab environment?
Reliability, safety, security, and alignment – is the AGI robust enough to deploy in the wild, with assurance that it will not fail in unusual circumstances, be subject to jailbreaks or other adversarial attacks, or deviate from its intended purpose?
Physicality – do we have robot bodies, and the software to control them, or is our AGI limited to browsing the web, sending email, and holding Zoom meetings? Are these bodies limited to basic tasks in controlled environments, or are they capable of performing surgery, assembling intricate machinery, navigating a construction site, delicate lab work, and supervising a daycare playground?
Adoption – given that the system is of practical use, is it in fact being widely used? Are regulators, slow adopters, consumer preferences, market friction, political pressure, or other factors standing in the way?
Note that many of these parameters will vary by use case; a system might offer world-class, fully independent, safe, reliable performance on some jobs, while being less ready for others.
Emergence Will Be A Long Process
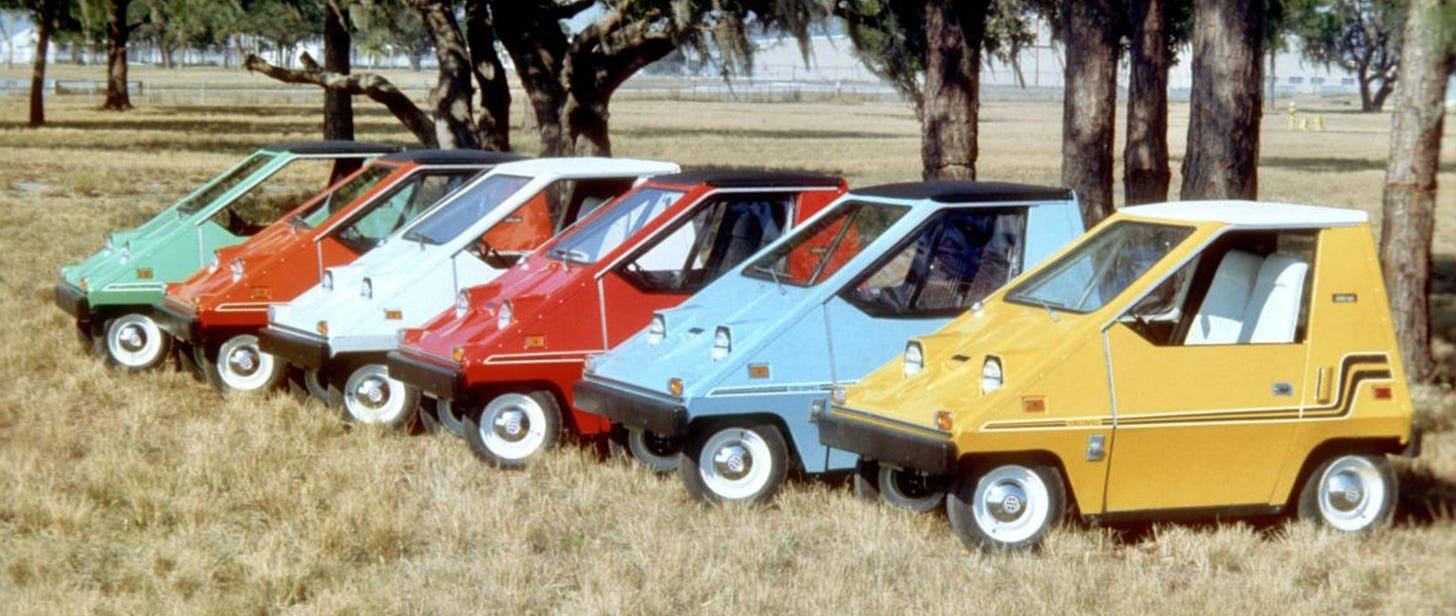
Each parameter listed in the previous section describes a hill that AGI will have to climb: from expensive to affordable, from mediocre to genius, and so forth. Here, I’ll say a few words about how long it might take to climb those hills.
Cost. The traditional parade of Moore’s Law cost reductions is drawing to a close, and the low-hanging fruit of improved circuit design for AI inference may also be running out. Transformative AGI by 2043 is <1% likely notes that the cost of GPU computation is declining at about 27% per year6, which is down considerably from the heady days of Moore’s Law. The primary source of cost reductions nowadays is algorithmic improvements. A paper from last December, Revisiting algorithmic progress, estimates that AI algorithms become twice as efficient every nine months.
On these figures, every two years, the overall cost to operate a given level of AI should fall by a factor of ten or so. At that rate, a lab-curiosity $1000 / hour AGI would take six years to reach the profoundly-transformative cost of $1 / hour. In practice it could be longer: there is no guarantee that the first demonstration of AGI would cost only $1000 / hour to operate, nor that improvements in algorithmic efficiency and hardware cost will continue at the present rate. So the journey from “AGI is possible at all” to “AGI is broadly affordable” will take years.
Availability – when competent, embodied AGIs become available at a reasonable cost, demand will skyrocket, requiring a massive increase in production of GPUs and other necessary components. Scaling semiconductor manufacturing capacity can take many years7.
Of course, this will depend on how much computing power the first AGIs need, and how quickly those requirements can be reduced. The open-source community has recently made impressive strides in obtaining near-GPT-level performance using models that are quite a bit smaller (and thus cheaper to operate) than GPT.
Quality – chess programs were playing at a passable amateur level by the late 1960s. The fateful Kasparov vs. Deep Blue match was held in 1997. Thus, it took about 30 years for chess AI to go from amateur to world champion. We should be open to the possibility that for some complex skills, it could take years for algorithms to progress from novice to expert.
Independence. The example of self-driving cars shows that going from a system that can handle 99% of the job to 100% of the job can take many years. (Of course, for many jobs a 99% solution can already be economically useful, if it fails gracefully.)
Training status. In some fields, people need decades to train up to expert level. The entire training process for GPT-4, on the other hand, seems to have been completed in roughly a year, perhaps less8. This may imply that advanced AIs will be able to learn specific tasks relatively quickly, but it could be that as we move to deeper skills, the training process stretches out to a more human-like time scale.
Real-world applicability. Quoting from Transformative AGI by 2043 is <1% likely once again:
In radiology, it’s been 11 years since the promising results of AlexNet on the ImageNet 2012 Challenge; and yet there’s been little impact to the radiologist job market.
They then present an extended quote from a radiology ML practitioner (page 20; original source here). Some highlights:
A large part of the radiologist job is communicating their findings with physicians, so if you are thinking about automating them away you also need to understand the complex interactions between them and different clinics, which often are unique.
Every hospital is a snowflake, data is held under lock and key, so your algorithm might not work in a bunch of hospitals. Worse, the imagenet datasets have such wildly different feature sets they don’t do much for pretraining for you.
This sort of thing might become less of an issue once we have true AGI, because in principle the system ought to be able to bridge these sort of gaps (communicating with physicians, learning to work with the unique systems in place at a given hospital) on its own, much as people do. But at a minimum, we may find that even AGIs, no matter how intelligent and knowledgable, need a certain amount of on-the-job training to become useful in a given workplace. And we may find that attempts to accelerate timelines or reduce cost by deploying narrower models (not full AGI) run into trouble in the messy real world.
Reliability, safety, security, and alignment. This seems like a wild card. In an earlier footnote, I mention that OpenAI reported spending 6 months aligning GPT-4, though it’s not clear whether this represents the entire safety and alignment effort, or just one phase. And the resulting alignment is notoriously not vary robust; hence the phenomenon of “jailbreaking”. We can tolerate jailbreaking today, because current models aren’t really powerful enough to be dangerous, but human-level AGIs will need to be held to a much higher standard.
It’s hard to predict how long it will take to safely align an AGI9. Presumably alignment techniques will have improved, but the bar will be much higher, and the models will be much more complex. So it could take much longer than GPT-4’s 6 months. In the worst case, we simply won’t know how to align the system, and the entire project might have to be put on hold until safety techniques advance.
Adoption. This is another wild card. The fact that many industries still rely on fax machines in 2023 should give us pause. If a business hasn’t yet upgraded from fax to email, how quickly can we expect them to move from people to AIs10?
From the discussion of radiology and ML quoted above:
Have you ever tried to make anything in healthcare? The entire system is optimized to avoid introducing any harm to patients - explaining the ramifications of that would take an entire book, but suffice to say even if you had an algorithm that could automate away radiologists I don’t even know if you could create a viable adoption strategy in the US regulatory environment.
For some applications, we might see adoption move quickly once the technology is ready. But in others, it could take decades.
To Project AGI Timelines, We Need To Map Out The Entire Process
As we’ve seen, the advent of AGI, from the first instance in a lab to broad deployment across the economy, will stretch out over a period of years; probably decades. The specific moment we’re interested in depends on which risk we’re concerned with, which development we’re anticipating, or which decision point we want to prepare for.
If you’re concerned about an AI-designed bioweapon wiping out human life, then a few expert-level bots in a single lab might be sufficient… though they’d have to understand real-world issues of synthesis and distribution, not just how to modify a DNA sequence for a theoretical increase in lethality.
If you’re wondering when AIs will start significantly accelerating their own development (“recursive self-improvement”), you’ll need an idea of how many virtual workers would be required (thousands? millions?), and how talented they need to be.
If you’re interested in the onset of massive changes to the overall economy, or worried about a robot uprising, then many millions of instances are required, and they probably need physical bodies.
The implication is that any useful estimate of AGI arrival should attempt to provide a map of the rollout, not a single date. Unfortunately, people often just talk about “the” date for AGI. This makes it hard to compare estimates and hard to use them to plan for AI risk. In the next few posts, I’ll try to work toward a map.
Thanks to David Glazer for feedback on this post. All errors and bad takes are of course my own.
If you’re interested in the topic of AI timelines, the paper is over 100 pages long, quite readable, and attempts hard quantitative analysis of a number of factors I hadn’t seen rigorously explored elsewhere.
Apparently they use this definition because it is (their interpretation of) a question posed by the Open Philanthropy AI Worldviews Contest.
A superhuman AGI at $1000 / hour might be much more than a curiosity, but that’s beyond the scope of this post.
Of course, market forces will connect availability with price: if demand exceeds supply, then the price will rise. However, for modeling purposes, I think it’s better to consider availability and cost (not price) separately.
Again, I’m not going to go into the (very real!) possibility of superhuman performance for now.
From the paper:
We estimate today’s rate of AI hardware progress by examining NVIDIA’s market-leading AI chips. Over the past ~3 years, from the A100 to the H100, NVIDIA delivered a ~2.1x improvement in FLOPS/$. This improvement rate of ~27%/yr is in line with the longer trend of GPU improvement from 2006 to 2021, estimated by Epoch AI to be ~26%/yr (but more realistically ~20%/yr if you exclude the unsustained rapid progress prior to 2008).
Transformative AGI by 2043 is <1% likely has a good discussion of the complexity of scaling semiconductor manufacturing. For instance:
The world’s most complex semiconductor tool, an ASML Twinscan NXE. It costs ~$200M, is the size of a bus, and contains hundreds of thousands of components. Just shipping it requires 40 freight containers, 20 trucks, and 3 cargo planes. As of late 2022, only ~150 have ever been manufactured. ASML is backlogged for years, with €33 billion in orders it cannot immediately fulfill. Over 100 EUV tools have been ordered, and ASML is producing them at a rate of ~15 per quarter (40–55 in 2022 and ~60 in 2023). The next generation version will be larger, more complex, more expensive (~$400M), and slower to produce (~20 in 2027–2028).
The exact numbers are not published, and it depends on which parts of the development process you count as “training”. I expect that OpenAI had folks working on the GPT-4 project well over a year before it was released. Once they had all of the issues worked out, the core training process is estimated to have taken between 3 and 6 months. OpenAI explicitly stated that they then spent 6 months “iteratively aligning GPT-4 using lessons from our adversarial testing program as well as ChatGPT”.
Some forms of AI risk apply even before the model is released; in particular, a sufficiently powerful model might be able to “escape confinement” while it is still being developed. Thus, we will likely need to incorporate safety and alignment into the core training process. It’s hard to say how that would impact the AI’s training schedule.
Admittedly, there may be a network effect at work in the stubborn longevity of the fax: if all of the players in a given market – say, finance or health care – exchange information using fax machines, it’s hard for any one company to move away from that. On the other hand, it’s not hard to imagine systemic effects holding back the adoption of AI in many fields; if your competitors are sending human sales reps to visit potential customers, do you dare send a robot?
I would definitely buy up "1-800-BIZ-BOTS" right now, it's going to be in hot demand in the next 30 years or so!