AI Creativity Is A Question of Quality, Not Novelty
It Actually Matters How Well The Bear Dances
ChatGPT and other large language models (LLMs) can spew forth essays and short stories by the bushel-load. How come none of them are of any real interest? OpenAI’s new “o1” model outscores PhDs on a test of expertise in chemistry, physics and biology. Why isn’t it generating novel scientific insights?
A popular explanation is that “AI can't create anything new.” The argument is that because these systems rely on human training data they are limited to generating remixes of existing work. But that’s an incorrect analysis. The actual impediments to AI creativity lie elsewhere. Understanding those impediments will help shed light on the prospects for those impediments to be removed, and allow us to evaluate the significance of advances like OpenAI’s latest model, o1.
Computers Create New Things All The Time
The idea that AI can’t create anything “new” does not stand up to scrutiny. Consider this snippet from The Policeman’s Beard is Half Constructed, a book written by a computer and published way back in 1984:
There once was a furry chilled stag
Had hairdryers which could not sag
They tripled and punted
And never quite grunted
And that's why they seemed like a fag.
There’s a problem with this limerick, but the problem isn’t a lack of novelty. The problem is that it’s crap.
Or consider this computer-generated content:
79BCE5AAFB9906BE63A1E5010B2ABACD133F9D59C758A1DE9B5F8EC1ED54A768
That’s a 256-bit random number. It may not be evidence of creativity, but it’s definitely new; I can guarantee that no one has ever written this exact sequence of letters and numbers before.
So, computers can create new things. Why, then, do we say that most work by computers today is not creative?
Creativity Requires Constraints
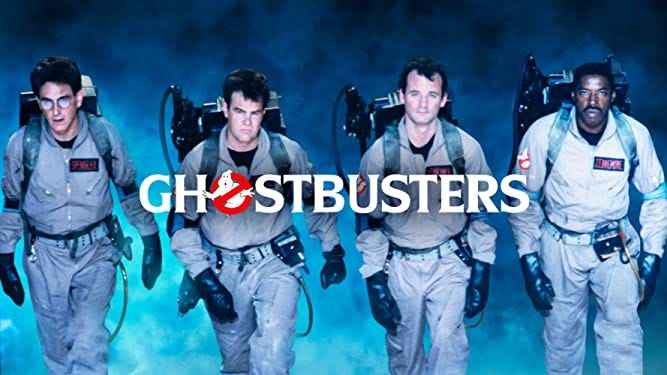
I think the reason that generating a random number doesn’t seem creative is because it’s too easy.
The Eiffel Tower was a great accomplishment, not because it was the first large structure made of iron, but because it achieved artistic beauty while simultaneously reaching literal new heights of engineering – being almost twice as tall as the previous record holder, the Washington Monument.
Ghostbusters was a great movie (yes, of course I mean the 1984 original) because it squeezed in laugh after laugh without disrupting the tension of the light supernatural-horror plot.
The last two seasons of Game of Thrones were widely criticized for inconsistencies and lazy plotting. Important developments were “unearned”. The term “unearned” is instructive – people disliked these seasons because the writers hadn’t done the work to satisfy the constraints of good storytelling.
For a piece of work to be considered creative, it must satisfy difficult constraints. That might mean characters acting in consistent ways, a thousand-foot iron structure supporting its own weight, or balancing comedy with a sense of supernatural danger.
When today’s LLMs fall down on creative tasks, it’s because they’re not capable of satisfying difficult constraints. A TV script generated by an LLM will be like late-season Game of Thrones: all the right elements are there, but they’re thrown together haphazardly, and the result doesn’t really work. The same thing happens today if you ask an LLM to design a complex piece of software, or explain a novel scientific phenomenon: it’ll generate something that looks right on the surface, but doesn’t stand up to inspection.
If “creativity” is actually about satisfying constraints, why do we associate it with novelty?
Novelty is Just Another Constraint
Deadpool is a great movie (fight me). It also grossed $783 million in ticket sales. If it were re-released today, it wouldn’t do nearly so well – because it’s no longer novel. The target audience has already seen it, and probably the sequels; the material is no longer fresh.
In 2024, it’s still possible to write a great snarky, self-referential action movie (The Fall Guy). But you have to do it differently. You need new ideas and new jokes. This is why genres get tapped out: it becomes harder and harder to find a new take.
To be entertaining, a piece of creative expression needs to be novel. That’s part of what makes a great book, movie, or composition such a creative achievement: not only does it need to be beautiful, engaging, coherent, and meaningful; it needs to do all that in a different way than what we’ve seen before. That additional constraint adds to the difficulty, and thus to our appreciation of the result.
So what does all this tell us about creativity and AI?
Judge AI By Its Ability to Satisfy Constraints
“The marvel is not that the bear dances well, but that the bear dances at all”. This is the lens through which we seem to view each new advance: the first instance of an AI accomplishing a new task is hailed as a major advance, even if (on reflection) it really did not perform the task very well.
It was remarkable when ChatGPT started generating essays on demand. And it was, perhaps, remarkable when Sakana – billed as “The AI Scientist” – started generating scientific papers without human input. But these things were remarkable primarily because they were novel; and novelty wears off. At some point, we want to watch good dancing, read insightful essays, get valuable research. By that standard, bears, GPT-4, and Sakana don’t measure up1.
By all accounts, the scientific papers produced by Sakana are dreck. It follows a cookie-cutter approach, mechanically tweaking an existing piece of AI software and evaluating the result. It contradicts itself, it hallucinates, it misses obvious prior work. Scott Alexander writes:
The creators - a Japanese startup with academic collaborators - try to defend their singing dog. They say its AI papers meet the bar to get accepted at the highly-regarded NeurIPS computer science conference. But in fact, the only judge was another AI, supposedly trained to review papers. ... In any case, if I’m understanding right, the AI reviewer only accepted one out of eighty papers by the AI scientist (and it’s not any of the ~dozen they’ve released publicly, which is suspicious).
All of this might be forgivable if Sakana also generated valuable insights, but I’ve seen no indication that this is the case. It generates new papers, but those papers don’t satisfy the constraints of high-quality research.
Meanwhile, Chess and Go AIs are indisputably creative2; human players have adopted new strategies first exhibited by computer players. In these narrow domains, specialized AIs have cleared the quality bar. Code authoring tools are steadily growing in sophistication and quality; you can debate whether they are “creative” but they are becoming increasingly useful (though still limited).
Rosie Campbell hit the nail on the head in a recent post:
So instead of asking "can AI be truly creative?," perhaps we should be asking "what can AI create?"
…
AI systems may soon be capable of discovering cures for cancer, creating novel pathogens, and even contributing to their own improvement, regardless of whether we label them "creative" or not. In the face of systems with such profound impacts, quibbling over definitions seems a little... uncreative.
The question of “what can AI create?” can be reframed as “in which problem domains is AI able to produce useful results?” This focuses our attention on the important question: what are the prospects for AI systems to start generating outputs that actually meet the constraints of the problem domain?
In a forthcoming post, I’ll share some thoughts on this question, and what the recently announced AlphaProof and o1 systems tell us about the likely pace of progress.
Thanks to Andrew Miller, Ben James, Dynomight, Julius Simonelli, Rob Tracinski, and Rosie Campbell for invaluable feedback and suggestions.
To be clear, GPT 4 has plenty of useful applications! But writing meh-quality essays isn’t near the top of the list.
In her recent post Can AI be truly creative?, Rosie Campbell notes:
…when DeepMind’s AlphaGo system defeated top Go player Lee Sedol in 2016, commentator Michael Redmond was taken aback by AlphaGo’s now-famous move 37:
"It's a creative move… It's something that I don't think I've seen in a top player's game."
Hey, you've probably already seen this guy, but just in case, he's been testing o1 on Math and Physics at a pretty serious level. I know you'll do your own deep dive/research, but thought you might find him interesting as well, as he takes the time to see how it succeeds and fails, in detail:
https://www.youtube.com/watch?v=2xYosqVjROc
https://www.youtube.com/watch?v=scOb0XCkWho
https://www.youtube.com/watch?v=a8QvnIAGjPA