Grounding the Conversation About AI
Organizing The (AI) World’s Disagreements And Making Them Universally Accessible and Constructive
Given how much I run my mouth on the topic of AI, you might think I understand the important issues. I only wish that were the case.
For instance, earlier this year, Microsoft and OpenAI reported that they have detected “state affiliated adversaries … using LLMs1 to augment cyberoperations”. Is this a big deal? Does it presage a tsunami of automated cyberattacks? I have no idea2.
I don’t know enough about the dynamics of cybersecurity to understand which tasks / skills are the key bottlenecks, so it’s difficult for me to judge the impact of any given advance.
This flavor of confusion – AIs are starting to become capable of X, but does X really matter? – pops up all over the place. Deepfakes can be used for political misinformation, but will that make things any worse than they already are? Some observers doubt it, arguing that the market for fake news is already saturated. Chatbots can write code, and there are claims this is revolutionizing developer productivity. But at the current level of capability, I’m very dubious.
Somehow no one gets around to answering these big-picture real-world questions: what are the key levers that determine the equilibrium in cybersecurity, or misinformation in politics, or the productivity of software engineers. This might explain why discussion of AI risks and benefits is often out of touch with the real world, leading to acrimony and bad takes.
It doesn’t have to be this way. We could be doing a much better job of making sense of the big questions around AI. The information we need is out there. But it’s scattered across too many different individuals, and no one is bringing them together.
Until now.
It's Hard To Make Sense By Yourself
If we aren’t working from a common understanding of how the world functions today, we’re going to have a tough time working together to make good decisions about how AI could and should affect the future. And in many cases, we don’t have that common understanding.
Consider the concern that AI could help a bad actor synthesize a dangerous virus. People have very different (mis)understandings of how difficult this would be, how close current AIs are to being able to help with the critical steps, and how serious the consequences might be.
I recently had occasion to dive into the topic; it was a frustrating experience. I’ve previously written about how difficult it is to make sense of academic writing. Scientific papers often omit important context, use terminology that seems straightforward but isn’t, or rely on over-simplified models of the real world. If you’re not an expert in the relevant specialty, you’re going to come away confused.
For instance, one paper suggests that it will soon be quite easy to synthesize a dangerous virus3:
Numerous independent advances in virology and biotechnology … have recently combined to render many viruses accessible to skilled individuals at a low cost. Step-by-step assembly protocols capable of producing infectious viruses … are widely available…
The typical advance … has required just … five years to be reproduced by undergraduates and individuals with moderate skills, and 12-13 years to become accessible to high school students and others with low skills and resources.
[emphasis added]
Another paper appears to say the opposite4:
…over the next 5–10 years, benchtop device advances may enable reliable synthesis of dsDNA up to 10,000 base pairs long. As a reference point, there are a few viral genomes that are shorter than 7,000 base pairs, but the vast majority are between 10,000 and 200,000 base pairs in length.
… It is possible to assemble shorter DNA fragments into these longer lengths, but the process requires skill and expertise and is not amenable to automation. For the foreseeable future—at least the next 10 years—it is unlikely that benchtop DNA synthesis devices will be able to automatically generate dsDNA at these greater lengths.
[emphasis added]
Cutting-edge labs have been able to routinely synthesize a variety of dangerous viruses for some time, so the first source implies that undergraduates could probably do it today, and high school students within the decade. The second source implies that this will remain quite difficult for “at least the next 10 years”.
It’s no wonder that people debating AI safety often seem to be working from very different ideas of how dangerous AI might be!
Misunderstandings Can Be Quite Profound
Not infrequently, seemingly reliable sources will repeat statistics that are wildly implausible, either because they were wrong at the source or because of a failure to capture important context.
Someone recently pointed me at an article in the Christian Science Monitor which cites “the OECD’s economists” as saying that:
People who write for a living and now rely on AI are about 50% more efficient. Computer coders are 60% more efficient.
As a statement about the world today, this is laughable. I presume the figure for computer coders was plucked from some study which measured the time to write a routine piece of standalone code. However, “computer coders” don’t spend that much time literally writing code5, and much of the code they write is not the sort that current AIs can reproduce. I don’t know anyone who writes software for a living and has personally experienced a significant productivity boost6. The practical scenario in which large productivity gains are seen today is when someone needs to write a straightforward, isolated piece of code in a domain that they are not personally experienced with. This doesn’t come up very often in practice, but it’s precisely the scenario that can be easily measured in a study.
My favorite silly claim comes up in discussions of AI and cybersecurity. I asked ChatGPT for the economic impact of cyberattacks, and it provided a figure of roughly $10 trillion per year. Which is insane. Ten trillion dollars per year is:
10% of the entire world economy
Well over $1000 per person
Close to $2000 per person who has access to the Internet
Twice the size of the global fossil fuel industry
That’s not a ChatGPT hallucination, it’s a collective Internet hallucination – pretty much all the sources I’ve found online use that figure. Does anyone really believe that cybercrime is twice the size of the fossil fuel industry? If not, then why is this figure routinely cited? I take it as a sign that most people have no real idea what is going on.
Many Heads Are Better Than One
I recently had the opportunity to work with the Center for AI Safety (CAIS) to produce brief reports on AI and biosecurity and AI and cybersecurity. These were topics I didn’t know much about. I started out by plowing through a bunch of papers and reports… and ran into all of the problems mentioned above: apparent contradictions, implausible claims, difficulty in understanding which facts were important.
Fortunately, I had another resource to lean on – the folks at CAIS helped me connect with multiple experts on each topic. When I was confused about something, I could ask. When I received contradictory input, I could point out the contradiction. When I circulated a draft report for review, I received corrections on points I hadn’t thought to question. With a day or two of work (spread over a longer calendar period), I accomplished more than I might have with months of solitary research.
Here are some (lightly edited) examples of invaluable feedback I received. Each of these directly addressed an incorrect or oversimplified understanding I’d been holding.
On the topic of excluding dangerous biological knowledge from LLM training data:
I disagree with that paper -- I don't think you could meaningfully separate those "concerning" types of experiments from the rest of virology. Also, the scientific community and NIH have all had this discussion already, and they identified a set of research (GOF research) as the type of research that might require additional oversight. It's a sensitive topic and [redacted] is outside the mainstream on this point.
On the harm that could result if someone synthesized a virus from a published genome:
This is true, yes, but very few known viruses synthesized today have pandemic potential due to existing immunity and their general epidemiology - think low R0.
E.g. someone could build SARS-CoV-2, but releasing it wouldn't have much of an impact considering the virus is already circulating.
Hence, there are only a handful of pathogens that, if constructed without significant modification, have any appreciable risk of causing 10s or 100s of thousands of deaths.
On the potential for nipping the next pandemic in the bud through strategic monitoring of viruses in wastewater:
Important to note that wastewater is promising but also won't be a magic bullet. The next HIV may not shed into stool or urine. Early detection should be layered with multiple systems - what about screening plasma banks? Sending swabs to people in the mail for a routine "infection survey"? Lots of promising possibilities.
This sort of targeted, “here’s what you missed when reading that source” information is invaluable in coming to a true understanding of a complex topic. And engaging with multiple experts, having different points of view, allows you to avoid blindly accepting one side of a controversial point.
Doing This For More Topics
There are so many complex questions around AI. How quickly will capabilities progress? How seriously should we take concerns around biosafety, cybersecurity, job loss, algorithmic bias, losing control of the machines? How feasible are advances in medicine, education, clean energy, general productivity? How might AI impact social media, elections, relationships, warfare? What would be the impact of various forms of regulation?
For each of these important questions (and many more), I think the path forward involves picking the brains of a diverse group. So I’m starting a project to do exactly that. We’ll pick a topic, convene a group, drill down on as many important, confusing, and/or contentious details as we can identify, and publish the results. Then do it again, and again, and again. There’s little danger of running out of topics.
We won’t strive for consensus; these topics involve too many unknowns, and too many subjective value judgements. Instead, the goal is to shed light on the full range of reasonable viewpoints, how those ideas relate to one another, and supporting arguments.
This may sound unwieldy. But I believe it can be done in a way that is accessible to casual readers, without oversimplifying. Each topic will start with an overview, and include links down to additional detail and supporting arguments. Organizing all of this will be a lot of work. But worth it.
Organizing The World’s Disagreements And Making Them Universally Accessible and Constructive
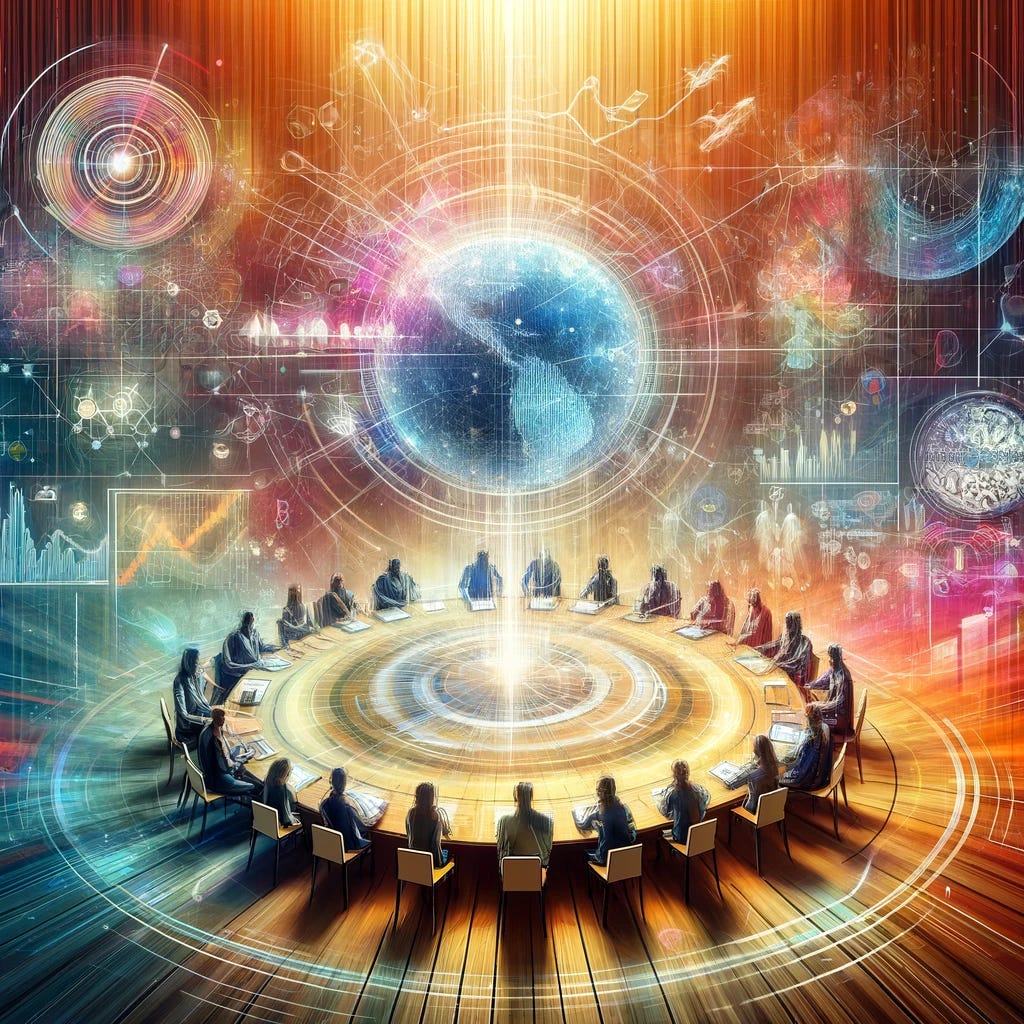
Google’s original mission statement was “to organize the world's information and make it universally accessible and useful”.
Every topic I’ve mentioned in this post is the subject of active online debate. Unfortunately, those debates don’t do much to advance the collective understanding. People talk past one another, miscommunicate, cling to out-of-date facts, and generally generate more heat than light. There is plenty of worthwhile material in the mix, but it’s difficult to locate. Like the pre-Google web, much of the discussion is hard to access and not very useful.
Through constructive engagement, we can use the diversity of viewpoints on AI to genuinely advance our collective understanding. But the work won’t stop when we publish a report. No matter how many people we can draw into a discussion, there will be ideas we failed to collect. No matter how hard we work on the writeup, we’ll sometimes fall short of clarity. And given the pace of developments in AI, anything we publish will almost immediately be out of date. To address this, we’ll publish living documents, forever open to feedback and updates.
My dream is for this to become a central hub where people go:
To discuss complex, speculative, and subjective topics around AI.
To understand those topics.
This should be a home for constructive conversation, a place where it’s fruitful to contribute ideas and knowledge. And it should be a trusted source for grounded analysis, presented in a way that is accessible to a broad audience.
This will be a little bit like Wikipedia, but unlike Wikipedia, we’ll often tackle speculative and subjective topics.
It will be a little bit like discussion forums such as Reddit, Hacker News, or LessWrong. But unlike those sites, we’ll actively recruit panels on topics of importance, and facilitate discussions that explore all of the nooks and crannies. We’ll maintain accessible summaries of each discussion, rather than expecting readers to dive into enormous discussion threads. And we’ll continuously update each topic over time.
There’s a reason this doesn’t currently exist: it will require a lot of effort – for moderation, analysis, and curation. I have thoughts for how to achieve this at scale, but that’s a topic for another day. We’ll start small.
Want To Get Involved?
If you’d like to follow along, there’s a WhatsApp group where I post occasional updates.
We’re convening our first panel, on the impact of AI on cybersecurity. I’d welcome suggestions for experienced folks to participate.
If there’s a topic you’d like to see explored, and you’re in a position to help recruit panelists, I would love to hear from you. Drop me a line at amistrongeryet@substack.com.
I.e. large language models like ChatGPT, though they’d more likely be using an “open” model that they can run on their own computers, such as Facebook’s Llama.
Well OK I have an idea: I suspect it’s not a big deal – yet. But that’s mostly just because, if it were a big deal, it’d probably be getting more press. My point is that I don’t know enough, for instance about how cybersecurity plays out on a day-to-day basis in the real world, to evaluate the question on the merits. Nor have I seen any informed commentary on the significance of this development.
From Delay, Detect, Defend: Preparing for a Future in which Thousands Can Release New Pandemics. The complete quote:
Numerous independent advances in virology and biotechnology, none of which is obviously threatening on its own, have recently combined to render many viruses accessible to skilled individuals at a low cost. Step-by-step assembly protocols capable of producing infectious viruses from a genome sequence and standard laboratory reagents are widely available, with particularly detailed and reliable instructions for influenzaviruses and coronaviruses, the families responsible for the last five respiratory pandemics. Such protocols, which are intended to obviate the requirement for “tacit knowledge” to successfully perform the experiment, have become increasingly common. The recent democratisation of biotechnology suggests that they have broadly succeeded: the typical advance made in a cutting-edge laboratory by individuals with doctorates has required just one year to be reproduced in other laboratories, three years to be adapted for use in other contexts, five years to be reproduced by undergraduates and individuals with moderate skills, and 12-13 years to become accessible to high school students and others with low skills and resources.
From Benchtop DNA Synthesis Devices: Capabilities, Biosecurity Implications, and Governance. One paragraph discusses benchtop synthesis capabilities and the length of viral genomes:
Current benchtop synthesis devices can reliably print DNA up to 200 bases in length, but it is very likely that newer devices will be able to reliably and automatically produce doublestranded DNA (dsDNA) up to approximately 5,000–7,000 base pairs in length within the next 2–5 years. Over the next 5–10 years, benchtop device advances may enable reliable synthesis of dsDNA up to 10,000 base pairs long. As a reference point, there are a few viral genomes that are shorter than 7,000 base pairs, but the vast majority are between 10,000 and 200,000 base pairs in length. Bacterial genomes are longer than 1 million base pairs.
Another adds context:
It is possible to assemble shorter DNA fragments into these longer lengths, but the process requires skill and expertise and is not amenable to automation. For the foreseeable future—at least the next 10 years—it is unlikely that benchtop DNA synthesis devices will be able to automatically generate dsDNA at these greater lengths.
From a recent interview with the CEO of a company that provides an AI tool that helps people write code:
[At] the largest companies, the amount of time that people spend only writing software is probably about 20%.
Based on my own experience, this figure seems plausible. The implication is that if AI tools could cut coding time in half, overall programmer productivity would only increase by about 10%.
I’m sure one could find examples of folks who claim that AI is making them much more productive. I’m just saying that there aren’t enough of them to intersect with my circle – which includes everyone I’ve met in a career building startups in Silicon Valley. I suspect that people who write code for a living and claim a big boost in productivity are either doing some very specific niche sort of work, or are greatly exaggerating (not necessarily lying, all sorts of cognitive biases could be coming into play).
One of the best use cases for AI coding assistants seems to be writing a simple, routine, isolated piece of code using a language or tool that you’re not experienced with. This is exactly what most people who are not professional programmers do when they want to take the pulse of AI coding assistants, so they come away with an unrepresentatively positive impression.
Steve Newman built a word processor with the last great platform shift to the Internet. Perhaps this is the next opportunity?
My guess is that you could work/automate towards something like the scenario you describe here:
> For each of these important questions (and many more), I think the path forward involves picking the brains of a diverse group. So I’m starting a project to do exactly that. We’ll pick a topic, convene a group, drill down on as many important, confusing, and/or contentious details as we can identify, and publish the results. Then do it again, and again, and again. There’s little danger of running out of topics.
Informed by an embedding-centric (rather than prompt-centric) approach as speculated on by Matt Webb https://interconnected.org/home/2024/05/31/camera
> We’re kinda getting accustomed to the idea of real-time translation (you speak in French, they hear English) although it is still mind-blowing that this will be shipping Real Soon Now with OpenAI’s GPT-4o.
> But real-time text hermeneutics, unearthing the hidden meaning of text and between texts? That’s wild.
> For instance, crossing this point with the previous one…
> What would it mean to listen to a politician speak on TV, and in real-time see a rhetorical manoeuvre that masks a persuasive bait and switch?
There was a startup called Crowdsmart who thought along similar lines worth checking out--Kim Polese and John Seely Brown were involved: https://www.crowdsmart.ai/